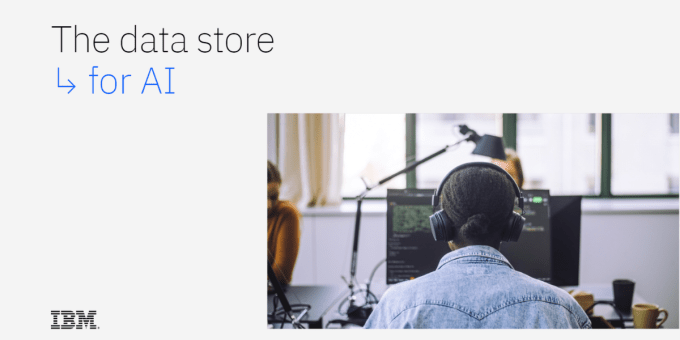
This e-book will examine the latest open data management solution for data and analytics leaders who want to significantly reduce cost, simplify data access and automate unified governance to scale AI. It’s time for the data lake house.
Data is at the center of every business. It keeps applications running, powers predictive insights and enables better experiences for customers and employees. But the full benefit of data is elusive because of the way that data is stored and accessed for analytics and AI.
You’re not alone if you rely on monolithic repositories with multiple data warehouses and data lakes, on premises and on cloud; 82% of organizations are inhibited by data silos.1 And it’s about to get worse: according to IDC, the amount of stored data is expected to grow 250% by 2025.
The data lake was supposed to fix all these issues; just land your data in a centralized place and process it. But it’s not so easy to update the lakes, properly catalog data or ensure good governance—and the skillsets required for these tasks are specific, rare and expensive. As a result, data lakes have proven costly to build and maintain. A data warehouse does offer high performance for processing terabytes of structured data. But warehouses can become expensive, too, especially for new and evolving workloads. Most organizations run analytics and AI workloads in ecosystems that are complex and cost inefficient. It’s time for a change.
Included in this Contents
- The current state of data architecture
- The data lakehouse defined
- Components of the architecture
- Cost optimization opportunities
- Analytics and data science enhancements
- BM watsonx.data
This article is posted at ibm.com
Please fill out the form to access the content